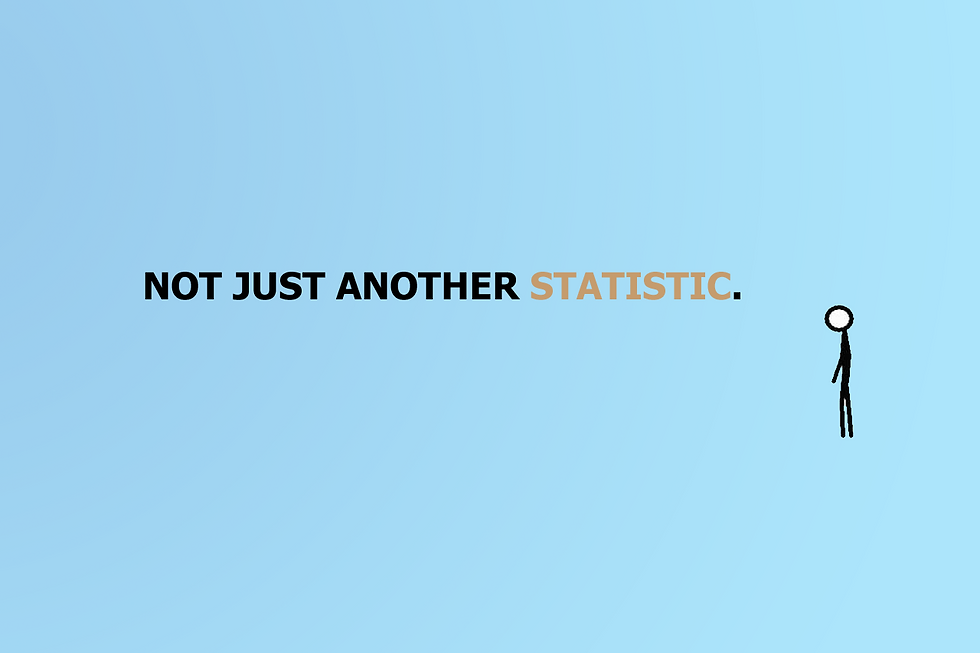
The portmanteau 'mathwashing' doesn't mean putting a stash of papers with math equations into a basin of water in an attempt to wash them off despite what the literal reading of the term may suggest.
Coined by Fred Benenson, the former Vice President of Data at Kickstarter, the term mathwashing can be thought of as "using math terms (algorithm, model, etc.) to paper over a more subjective reality." [1]
Mathwashing is probably reflective of a larger phenomenon where numbers tend to be seen as a more legitimate source of information or evidence despite their potential to be less objective due to the hidden bias, subjective preferences or direct involvement of those involved in the production of such data or the creation of such algorithms/models/data driven products.
This is in spite of any intention on the part of those that are involved in the creation and use of these numbers.
In Benenson's own words to Technical.ly:
Algorithm and data driven products will always reflect the design choices of the humans who built them, and it’s irresponsible to assume otherwise...
.....There’s no such thing as perfect data, and if you collect data incorrectly, no math can prevent you from making a bad or dangerous product. In other words, anything we build using data is going to reflect the biases and decisions we make when collecting that data.
An often mentioned example of mathwashing was the drama surrounding Facebook's Trending Topics section.

In May 2016, an article by Gizmodo interviewing former Facebook news curators (contractors hired by Facebook to curate its Trending Topics section) pulled aside the curtain to reveal the controversial inner workings of the Trending Topics section.
The crux of the issue was that despite public perception that the Trending Topics lists were determined by a value-neutral algorithm, the reality was in fact much more different.
Humans were heavily involved in the process from filtering news topics to editing topic titles and descriptions (amongst other things) while their involvement and importance in the process were kept secret from the public.
Alongside this reveal was a follow-up article where allegations were made by former members of Facebook's news team that conservative topics were being routinely suppressed from appearing in the 'highly-influential section, even though they were organically trending among the site’s users.'
Facebook quickly responded with an official statement from Justin Osofsky, VP Global Operations and a few words from Facebook executive, Tom Stocky and Mark Zuckerberg .
Justin Osofsky:
First and foremost, the algorithm that surfaces topics eligible for review optimizes for popularity and frequency on Facebook and whether it is a real world event — and does not consider perspective or politics.
Second, we have a series of checks and balances in place to help surface the most important popular stories, regardless of where they fall on the ideological spectrum, as well as to eliminate noise that does not relate to a current newsworthy event but might otherwise be surfaced through our algorithm.
Facebook does not allow or advise our reviewers to discriminate against sources of any political origin, period.
It was also reported by the Guardian that:
"Former employees who worked in Facebook’s news organization said that they did not agree with the Gizmodo report on Monday alleging partisan misconduct on the part of the social network. They did admit the presence of human judgment in part because the company’s algorithm did not always create the best possible mix of news."
However, Facebook's leaked guidelines for reviewing trends, which was published by the Guardian later in the week showed certain inconsistencies between the matters contained in the leaked documents and what was said by Facebook in its response to the allegations.
Whether the allegations that were made against Facebook were true or not, Facebook's subsequent act of laying off the entire editorial staff of the Trending team that it hired, opting to 'no longer employ humans to write descriptions for items in its Trending section' in a bid to make the Trending feature more automated, was perhaps not the best move.
Barely two days from the announcement, 'the fully automated Facebook trending module pushed out a false story about Fox News host Megyn Kelly, a controversial piece about a comedian’s four-letter word attack on rightwing pundit Ann Coulter, and links to an article about a video of a man masturbating with a McDonald’s chicken sandwich.' [2]
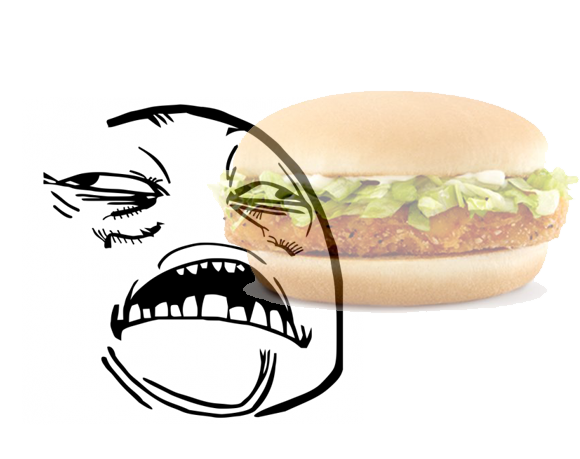
Truly newsworthy.
More than half a year since the change in algorithm, the Trending Topics section is still not free from problems that it had faced in the early weeks of the algorithm change with conspiracy theories and lies sneaking its way into the trending section of Facebook.
Ironically enough, Facebook's response in drastically decreasing reliance on human judgment had contributed to an increase in its unintended endorsement of fake and irrelevant news through its Trending Topics section.
Ok, so why tell me any of this?
Because if there are lessons to be learnt from the Facebook situation, they would be the following:
Drastically removing human judgment from the equation is not the way to go. A purely objective algorithm/model with no human involvement may not exactly be the best method in achieving Facebook's desired outcome which was 'to surface the major conversations happening on Facebook'. [3] Although the algorithm in Facebook's situation literally did as it was asked to do by providing what topics were trending, it failed to filter out news/topics that were false, unreliable or less noteworthy. Even if it did, it would have reflected the subjective preferences of its creator in doing so. Funnily enough, the algorithm had problems precisely because it objectively reflected the honest truth about Facebook's users and the quality of conversations on the platform.
The subjectivity that factors into these algorithms should have been embraced and not kept from the public. While human judgment will continue to be needed in the interpretation of data, Facebook's actions of being discreet with the involvement of humans in the early years of the Trending Topics section unsurprisingly caused a few concerns seeing as how Facebook is and was one of the major platforms in which internet users share and receives news on a daily basis.
It is important to note that Facebook's previous approach and method (prior to May 2016) is not a unique one and could perhaps be seen in many other algorithms or data driven products used by other companies or even governments. This is because human involvement is perhaps to a certain extent inevitable due to the limits of modern day technology.
While human involvement is inevitable, humans themselves are prone to error and we will dive a little further, taking a look at some of the problems or weaknesses of a data-driven approach.
Two key stages of such an approach are:
Data interpretation
Data collection
The problems or weaknesses of each each will be looked at respectively.
DATA INTERPRETATION
It comes as no surprise that even those that are trained to interpret data and understand its shortcomings, are prone to making a fews mistakes themselves.
Taking the quick quiz below might surprise you as to how easy data can be misinterpreted. (The quiz is based on this linked publication on statistical genetics)
Consider the following statement then answer the question below:
“There is a blood stain at the crime scene. The chance of observing this type if the blood came from someone other than the suspect is 1 in 100.”
Are the following statements true or false?
“The chance that the blood type came from someone else is 1 in 100, therefore there is a 99% chance that it came from the suspect.”
“The evidence is 100 times more probable if the suspect left the crime stain than if some unknown person left it.”
Answer:
False.
True.
The test above demonstrates a common mistake known as the prosecutor's fallacy. (there's also another common mistake called the defense attorney's fallacy but we won't be addressing that in this blog post)
The first statement is false because the original statement does not state that the chance that the blood type came from someone else is 1 in 100, it states instead that the chance that the blood type came from someone else if the blood stain was left by the suspect is 1 in 100. It is conditional upon the fact that the blood stain was left by the suspect. The probability of whether the blood stain was left by the suspect is not 99/100 and will be dependent on other available evidence and not by the presence of blood stain itself.
The high chance of mistakes in interpretation occuring when it comes to statistical genetics have been a cause for concern and is also one of the reasons why it is important to note that DNA evidence is circumstantial.
It is quite frightening to think how a slight change in the way statistical results are phrased can cause quite a big difference in interpretation and such mistakes are often more commonplace than one would think.
The problem is further amplified by the careless reporting of certain news sites that spread incorrect interpretations of data to the public.
DATA COLLECTION
This blog post will be focusing more on the common weaknesses of the methods used to collect data, more specifically problems that are inherent in the data collection process and the method adopted.
Such weaknesses in the data collection process aren't too obvious as they tend to remain hidden under the often-complex methods used to obtain such data, much to the detriment of the layperson who most likely would not have the time nor the interest to analyse or critically think about the data and its potential defects.
Weaknesses in the data collection process are commonly caused by:
Ignoring qualitative data
To make this more relatable, we will use an example:
You have a hypothesis that the number of shoes owned by people name Bob are directly caused by their level of happiness.

Bob and shoes
We can count how many shoes each person named Bob in the neighbourhood has (quantitative data) but if we don't ask each Bob in the neighbourhood why he has that amount of shoes (hence getting some qualitative data), we would be left to speculate as to the reasons each Bob has a certain amount of shoes.
We would not be able to ascertain the reasons that those Bobs got those shoes, thus failing to prove the hypothesis.
Reliance on data which is solely quantitative but not qualitative won't paint the full picture. This is the problem with the large amounts of data that is gathered by Google, Facebook, Twitter and so on.
(Ignoring Occam's razor) While Google can show that Amy has google searched 'cute cats' 10 times in the past hour, Google can't tell if:
a) Amy is planning to buy a cat for her sister, Lucy.
b) Amy's phone is being used by Lucy who is fond of cats.
c) Amy likes cute cats
Absence of qualitative data that may be obtained by asking Amy why she googled 'cute cats' a weakness in the data.
Failure to differentiate cause and effect
Now, assume that you try to ascertain the income level of each Bob in the neighbourhood as an indicator of their level of happiness.
You notice that the number of shoes that each Bob has seem to increase with the level of their income.
This means that the number of shoes that each Bob has is directly influenced by his level of happiness, right?
Nope. This is a critical thinking flaw known as confusing cause and effect.
By making such an assumption, we are jumping the gun by assuming that the level of a person's happiness is directly influenced by their income level when this may not exactly be true.
Even if it was, we still can't establish a causal relationship between the number of shoes that each Bob has and their level of happiness.
It could be the other way around, in that, the more shoes Bob has, the more happier he gets.
Too many variables at play
When it comes to data collection, there's no such thing as too many variables to consider and missing out on one or two important factors may be the key difference as to whether the hypothesis is proven to be true.
For example:
Bob #1 could instead be buying more shoes because he has an abnormally sharp foot rather than because he is more happy.
Bob #2 could be buying more shoes because he is getting more happy but also because he wants to look attractive.
The amount of variables to consider can be huge but failure to account for most of them may lead to inaccurate data.
Small sample size
While we may know the amount of shoes that Bobs in the neighbourhood have, we are still a long way from seeing if our hypothesis is true.
The sample size of our data is just too small and may not be reflective of Bobs throughout the world (a scope which our hypothesis ambitiously tries to cover).
Human subjectivity and lies
If we look back at Amy's situation with Google, Google may ask Amy the reason for her searching 'cute cats' but the situation is not as straightforward as that.
This is because Amy may lie about her intentions.
Consider another situation in which a group of students are surveyed. There are various potential problems to consider.
Who decides the questions to be asked? Will the framing of the question affect the answers that are given, hence, jeopardising the results obtained?
There is also the possibility that answers given to the survey may not exactly be true despite the intention of those participating in the survey.
People may have a bias account of their own experiences or attributes and their answers in the survey may not exactly be reliable. This could be supported by what is known as the Hawthorne Effect (a phenomenon where participants in behavioural studies change their behaviour or performance in response to being observed by the individual conducting the study).
A CAREFUL APPROACH
The mathematician searches around the lamppost on his hands and knees. “What are you looking for?” a bystander asks.
“My keys, I dropped them as I was leaving the bar” comes the reply. The bystander looks over his shoulder, “But the bar is back that way”, he says, pointing into the distance.
“Yeah, I know, but the light is much better here” the mathematician replies.
Data would perhaps be better served as a tool rather than a means in achieving a desired outcome. The joke above regarding the mathematician encapsulates perfectly the problem with over reliance on data.
Add in the potential problems with data interpretation and data collection that we have just looked at and it would be safe to say that there needs to be a more careful approach when it comes to data. The effects of being reckless with collecting, interpreting, and using data can be severe.
While I couldn't care less if some company sought to rely on data to maximise their profits, I do, however, care about the larger impact of data in important fields such as medical science where correcting a mistake may take many years, costing lives in the process.
For me, human rights is also an area in which I believe data should be taken very seriously.
Data has undeniably played an important role in human rights struggles, a key example being the civil rights movement in America during the 60's where key population figures based on race assisted in the implementation of the Civil Rights Act.
However, ignoring the potential flaws of data and the danger data may pose if misinterpreted, is to perhaps willingly turn a blind eye to a man that means you harm.
Human rights struggles are often not without its perpetrators and it would not be too far-fetched to suggest that these perpetrators would, if given the opportunity, deliberately twist words or data to fit their agenda. [4]
Once the misinterpreted data has been normalised by these perpetrators who may use it to justify the systemic oppression that they support (probably because their payroll and status, whether of a religious or social one, is dependent on their compliance with the system), it will be difficult to turn back the clock and undo the harm.
When it comes to human rights, there are many issues to consider.
Who is gathering the data and presenting it? Are they truly capable and independent? What is the quality level of this data?
There is the added problem of trying to distill people's suffering into numbers. This arguably desensitises the plight of those that are deprived of human rights because numbers may not be able to fully represent the suffering of these individuals.
Can these numbers, for example, represent the deprivation of liberty, mental suffering, and shame that a wrongfully convicted political prisoner experienced in his time in prison?
Those arguing that the opinions of the majority should trump over the opinions of the minority when it comes to human rights are perhaps misguided in their arguments.
The fundamental rights of a human being should not be dependent on the opinion of the majority. The events of WWII should have been enough to prove this. It is often the minority that are the ones being persecuted due to some perceived inherent trait or factor (despite how illusory these traits or factors tend to be) that distinguishes them from the majority (e.g ethnic minority or indigenous groups).
If the reason why data is being sought is to see if action is required to remedy a potential abuse of rights situation, the question that must be asked is: what percentage would compel action to be taken? 20%? 10%?
If 50 citizens in the population of 1 million were found to be tortured by Government operatives in a secret mission not previously known to the public and not experienced by almost 99% of the population, would action calling for the prohibition of torture be unqualified because 50 is not a good enough number?
Can we really reduce the suffering of these 50 people to the statistical percentage of 0.00005% and call it negligible, thus capable of being ignored?
If the effect on one individual should be ignored, why have independent public inquiries over the death of Stephen Lawrence, which paved the way for police reform in the UK?
How about a single person, then? Should Teoh Beng Hock's mysterious death be ignored because it was a one-off incident involving the MACC?
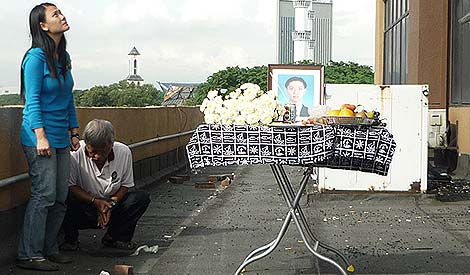
Photo courtesy of Lucia Lai (http://lucialai.org/2010/07/17/fighting-for-justice-for-teoh-beng-hock-continues/)
While only one event may get the media spotlight, it doesn't necessarily mean that there aren't others that haven't suffered the same fate or might suffer the same fate in the future.
As such, there is a need for a careful approach when it comes to data whether it is sought to be relied on in situations involving human rights or other situations that invoke deeper concerns involving fairness and justice.
Despite the potential flaws in its collection and interpretation, data is nonetheless an important component in decision-making but as highlighted repeatedly in this blog post, a careful approach is needed and it ought to be remembered that data often isn't really worth worshiping.
To quote an article by Inside Intercom:
"If Apple were driven by data points, they would release a €400 netbook or shut down their Genius Bar after years of no activity. If Ryanair were customer driven they’d remove all their sneaky fees & charges. If Zappos were driven by margins, they’d abandon their generous returns policy.
They’re all just perspectives.
Just because data is objective, it doesn’t mean that it guides you to the right decision. Just because it’s precise, it doesn’t follow that it’s valuable."
Comments